Data Cleansing in a Data Quality Management Framework
What is Data Cleansing?
The cleansing and validation process is iterative to continuously maintain and improve the quality of data to meet organisational or end-user expectations or thresholds. Metrics are calculated by the data validation framework and displayed for auditing and reporting. Dashboards are used to monitor data quality with connected notifications which are triggered if validation or threshold rules are breached.
Duplication
The removal of data that does not belong in the data set safeguards the golden copy data in the data warehouse. Data cleansing becomes particularly important when combining multiple data sources as there is a greater risk of duplication. Duplication can occur when combining different data sources, from multiple locations including data residing on the web or multiple departments within an organisation.
Removing outliers
Outliers caused by incorrect data entry or data mapping can skew data analysis and resultant insights. Removal or isolation of outliers based on data thresholds optimises data quality but also provides useful statistics. A small percentage of outliers may not require additional investigation; however, larger percentages may necessitate a more in-depth investigation and the shifting of thresholds. Any outlier can be added into data sets if they are valid data points. In the case of duplicates and bearing in mind the process for outliers, rules can be set to choose the best value for each data attribute.
Missing data and validity checks
Data sets with missing data can also be cleansed to ensure the stability and consistency of the data set. Rules can be set to check missing data based on relational data rules. That is if a unit is present the price of the unit must be present. It is possible to supplement data sets based on relational data rules. For example, if unique values always describe a particular data property they can be filled in for certain properties. Data can be linked based on the existence of unique identifiers. The line between data quality, where data is reliable and accurate and data integrity where data sets are complete, accurate and consistent are often intertwined.
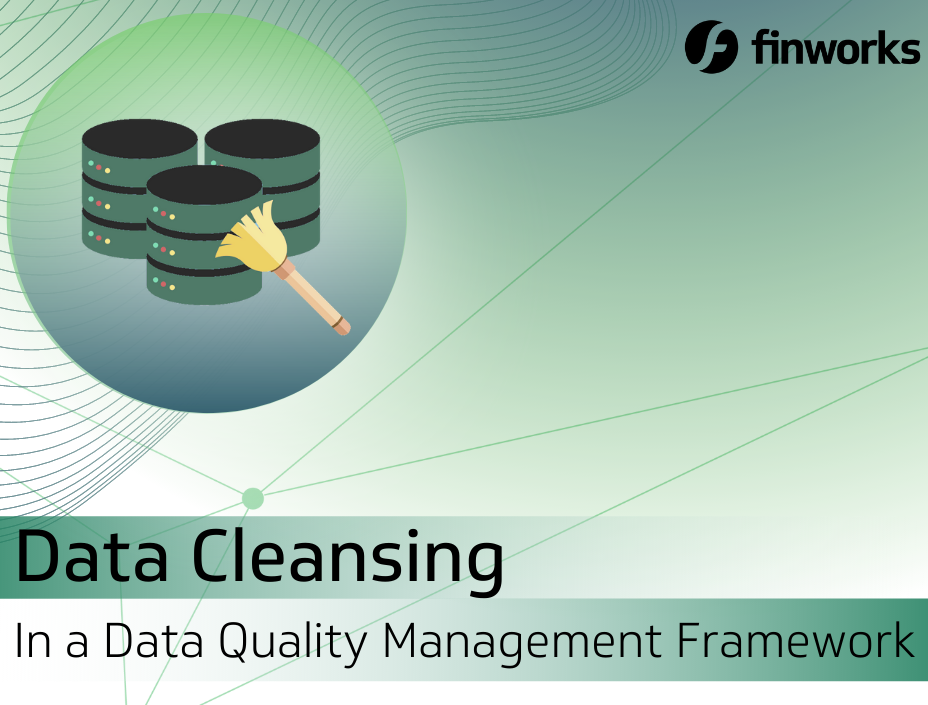
Data Quality Management Framework
Data cleansing is a crucial part of a data quality management framework. The framework applies every time data is input, for example, when data is input from multiple sources including key data sources, commercial data suppliers, third-party data and internal sources. The framework concept uses data quality requirements, attributes, dimensions, calculations, and rules.
- The first step in the data quality management framework is quality control where the data is converted to standard formats with technical data quality checks.
- The incoming data set is compared to the past set to get temporal insights on data quality.
- If there are multiple sources of the same information cleansing can be based on reliability weightings so data from more reliable sources is retained.
- After duplicates are filtered, automated processing of the data occurs through cleansing workflows.
- Validity checks choose the best (most reliable) value for each data point if sources are contradictory. That is, if the data does not match, the most data source will be selected.
- Virtual data attributes are calculated to derive missing data or complete data sets for analysis
- Consistency checks measure the likelihood of data being accurate
The result of the data quality management framework is the “golden copy” of the data which is the most complete and reliable data set based on the data inputs. The data is stored in the data warehouse and available for business intelligence tools or dissemination to downstream systems. With analytics, the need for data quality can vary widely by use case. The definition of quality depends on the use case, and each use case has unique needs for data accuracy, precision, timeliness, and completeness.
About Finworks
Finworks has years of experience in creating and implementing data quality frameworks for clients. Finworks Data Fabric ensures your data is fit and ready to use when and where it is needed and in the correct form for onward consumption.