Tech Spotlight:
An interview with a Data Expert on Building World-Class Data Science to Drive Organisational Success
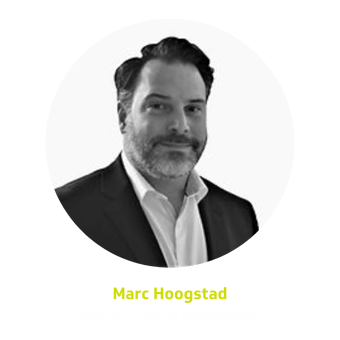
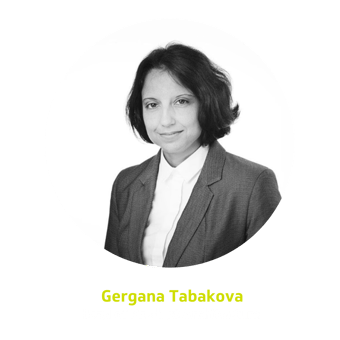
Today, Marc Hoogstad, Head of Product Management and Gergana Tabakova, Head of Product Architecture, will discuss the key components of a world-class data science function and how organisations can leverage data to create value.
How would you describe a world-class data science function?
Marc: Data science is the study of data to extract meaningful insights for organisations. Overall, the goal of a data science function is to leverage data to drive strategic decision-making, improve processes, and create value and data products for the organisation.
How would you describe a world class data science function and what are the main components?
Gergana: First of all, data science is quite a hot topic with our clients, especially after migrating to the cloud. On premises (on-prem) it is difficult to have a platform that is easily integrated with their systems, their data and their security. Everything was disconnected.
Now when everything is very much connected on cloud, clients are looking to accelerate their data science opportunities by finding new insights from their data and new data products. For example, a client is looking to generate a new statistical product that they can publish. Clients want to enrich their data and onboard new data sources. With data science they can see how these data sources may impact their data even before loading the data fully.
What are the main components of data science?
Gergana: The main components of data science include:
1. Onboarding and integrationThe data management platform is the way to integrate the data sources. It is possible to easily collect and onboard many more data sources, which we don’t want to process but which can be used for data analysis. The data management platform should offer functionality for easily onboarding data from various data sources and still stay connected with the golden copy of the data and all the other data which is stored together.
2. FlexibilityThe data management platform needs to be flexible in terms of connectivity in terms of pulling and pushing various formats and connecting to all kinds of other systems to pull new data which they can use for data science. Data scientists need a platform to allow them to transform this data. If they are to combine these new data sources with their existing data then they need to transform data to change codes, format, and scales. So, the data management platform must allow them to clean the data without additional development. The data needs to be pre-processed so that they can do further analysis. Overall, data scientists use the data management platform to collect all kinds of additional data which they will analyse together with their existing data.
3. Modelling
The next stage is model development. The data management platform should allow data science professionals to write data science and machine learning code with a single sign on so that it seamlessly integrates a variety of data sources. The platform should also offer functionality for reading various formats like files, databases, or streaming sources. In order to apply machine learning algorithms, the data is read and then experimented with because part of data science machine learning is experimenting with models and model parameters.
Marc: Is the goal to try to use AI or machine learning in model making?
Gergana: Yes, machine learning is used plus they use standard data transformations. They primarily use Python or R, at least our clients, so they want the platform to support Python or R but be able to download and use new libraries whenever there is a new library available. Data scientists will feel comfortable with the data management platform when all the tools that they need for their role are available to them. So, the platform should not limit them. Instead, the platform should help them to use whatever they are comfortable with.
4. Scalability
Data science also requires that the platform should be scalable. Machine learning especially requires significant CPU power and if they want to apply to large data, they usually ask for large machine resources. It means that the platform should be scalable. They may run their models for a day or two and then they don't need this power it should be possible to scale it up and down; up so that they can perform their work and down whenever they don't need the resources. This is easier to do in the cloud.
5. Publishing
Publishing is the next requirement. How can reports or models be published so that it is seen by everyone in the organisation. In addition, how can other BI tools be integrated, especially if the report can be reproduced and the data can be published.
6. Security and backups
From security perspective, everything should be integrated so no tools are isolated.
The platform should ensure that it is secure from data loss and code loss. The results should be backed up including temporary test results. Overall, the code is backed up and the environment is backed up as part of the security of the platform.
7. Confidential data
Production data is usually highly confidential, and data scientists need to be able to experiment freely. The data management platform should allow them to perform data masking or to anonymize the data. Data masking allows the use of production data for tests without the worry that it is highly confidential data that is being used.
Marc: Yes, because it's not always possible just to limit research to data that doesn't give away any personal information. Data science can only go so far with data without any identifying information. A data management platform has to be able to deal with data that has some privacy aspects, but you can pseudonymise it or anonymise it.
How is Finworks differentiate from other providers?
Marc: Are there any aspects that differentiate what we do for our clients that other providers might not do?
Gergana: What we try to do is to make sure that all the data science as a tool, as a concept, as an operation is well integrated within the system itself. The system allows data science to happen in the easiest and most secure way for the customer by allowing them to automate data pre-processing, data masking and not to worry that the data is confidential, not to worry that they need hard code passwords for each data source so that everything is integrated within the overall solution.
Our clients know that their data and their code is also secure. The platform takes care of everything so that they can only focus on writing the models, testing the models, and tuning parameters.
Marc: That's really important. So, they're not wasting their time doing other things, they're just focusing their time on the actual data science. And I suppose if everything is integrated, then if they do find one data source, for example, is superior or they know that they want in the future to include that data source into the main data management functionality, then it will be quite easy to move it from the experimental side into the operational side. Is that right?
Gergana: Yes, it is.
Marc Hoogstad: How often are data sources added? I know they have their main sources of data that probably hasn't changed a lot, but have you seen changes based on the data science work or even based on moving to the cloud?
Gergana: Right now, they're trying to onboard several such data sources. They way they do it is that they copy a file onto the data science platform and start experimenting and predicting the impact the data quality and compare data coverage this versus the existing one. In addition, it is necessary to define how the data should be mapped because they can be completely different data models. They also have to analyse how the data are stored versus how the data source sends the data. So yes, they're currently doing that.
Marc Hoogstad: So that ties into the value that they're able to get from data science. So, they're able to get better data coverage by including other sources of data to get a better quality data set for downstream processing. Is that the value that they're getting from data science activity and is there anything else that you know of?
Gergana: This is currently the core of it. Better data coverage, better data quality.
They started experiment with data models and getting more insights from the data. But it's an early stage because they have just started. So, it will take a couple of months of experiments until we hear about some results.
Future trends of data science.
Marc: The middle to long-term goal is to get better insights from their data by understanding how they can use that data to get the best value. What do you see as future trends?
They're improving data quality; they're improving coverage they're trying to get better actionable insights from their data. What are the future trends with their data science activity?
Gergana: The goals are improving the coverage, improving the quality, and having a bigger data set. The long-term goal is to use the data more widely within the organisation. Currently, it is used for one specific purpose. If the data set grows, then it can validate many more inputs within the system. It can integrate better with the rest of the data within the client data systems. The goal is for many more downstream systems to have access to and use the data.
Marc: Yes. So, by joining up everything you make data more available for people overall.
Gergana: Yes.
Marc: We talked about the feature of combining data science with the data management platform and why that's important. But we haven't talked about speed. Have you seen the benefits in terms of processing or the ability to review and model data either because of the optimised platform or because of being on the cloud or both? Is being able to quickly analyse big sets of data important?
Gergana: It is very important because with their big data volumes, they really need machine power to be able to process the data or apply whatever model to the data. On-prem it wasn't possible, but now in the cloud, it is possible that they can use the platform for let's say 12 hours with a high number of cores and memory to run their models and then they don't need it for the next couple of days. This keeps their cost manageable at the same time they get performance which wasn't possible to get before on-premises. They have access to the most powerful resources, but they only pay for them per use; they use them and then they release them.
Marc: This gives them that flexibility to use it whenever they need to and then, but also to have it more powerful as well, that which is the best of both worlds, isn't it?
Thank you for the detailed and insightful answers. It was a pleasure learning more about this topic from you.
Ready to Get Started? Get in Touch Today.
Ready to put these data science insights into action? Take the first step towards transforming your organisation with Finworks. Our team of data experts is ready to help you unleash the power of your data and achieve unparalleled results. Contact us and schedule a free consultation with our specialists. Let's work together to create a tailored data strategy to propel your business to new heights!