Data Enhancement to Refine, Improve and Enhance Data
What is data enrichment?
Data enrichment is the process of enhancing existing information by supplementing missing or incomplete data. The data enrichment phase modifies the data to reduce its amount or improve the information content. Data enrichment is defined as a type of data integration, filling in missing details to see a problem and its solution clearer. Data enrichment is achieved by appending one or more data sets with other attributes and values from different data collections including internal or external sources. The process begins with the quality of the existing data. If the existing information is incorrect or too incomplete, it may be impossible to match to a reference source to supplement what is missing.
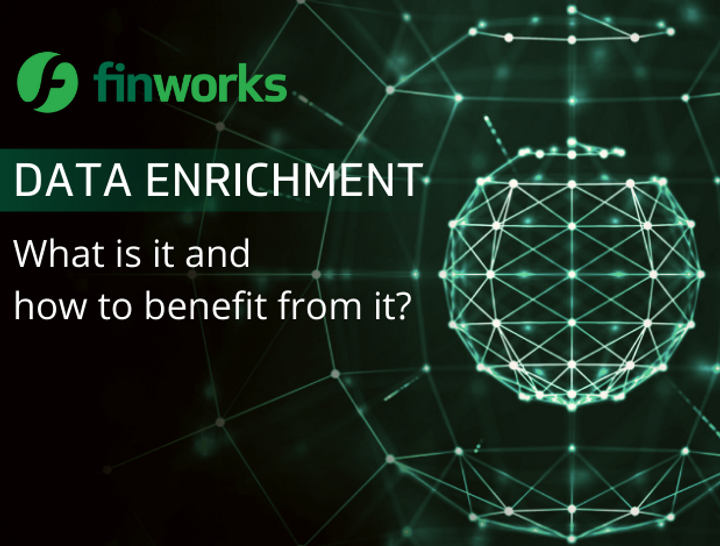
Enrichment as part of data quality measures
Before enriching your data, it is important that it is clear what is needed and how it is going to be used. The data enrichment process takes place after the assessment of the quality, completeness, and accuracy of your existing data. Data quality is established as part of data discovery, ingestion and transformation processes. Often it involves a combination of business rules developed in ETL/ELT programs and it is measurable process.
Data transformation is implemented as part of ETL/ELT processes as defined by business requirements by the user teams. Data transformation uses master data and metadata program outputs for referential data processing and data standardization. Additionally, it includes auditing and traceability framework components.
All the technologies used in the processes described above have a database, a user interface for managing data, rules and definitions, and reports available on the processing of each component and its associated metrics.
If the data application requires accuracy and precision in the data, attempting to use unacceptable data will introduce a risk that the results may not be trustworthy. If incorrect values are an impediment to the analysis and making changes does not significantly alter the data from its original form other than in a positive and expected way, data enhancement and enrichment may be important and valuable part of the data quality process. Enrichment requires taking other data sources and making it compatible with existing databases, ready to support activities like producing reports or providing startling business insights.
It is necessary to understand and plan for data enrichment as it must serve a business purpose. When matching data to another source, there is always the risk that the match will not be accurate. It is important then to obtain or produce an automated score representing the confidence level with the match. The organisation should determine what is acceptable for their business or process. An example of this is:
- Higher range: For example, 80 percent and above, where matches are automatically accepted
- Middle range: For example, between 60 and 80 percent, where matches must be manually analysed to determine if they are good or not
- Lower range: For example, 60 percent and below, where matches are automatically refuse
Once a match is deemed correct, the additional information provided by the reference source can be used to enrich the existing data. Enriched data describes valuable data relevant to the problem and situation at hand. Enrichment should serve a purpose, to segment the data or enhance analysis to maximise the ROI from the data enrichment activities.
Data Enrichment helps standardise data to suit data quality needs to create a refined, improved, and enhanced data grouping. Data enrichment isn’t a task that is done once in an organization. It’s an ongoing process to ensure that data remains viable and has value for your organization. With that said, it’s crucial to make sure data is up to date and is continuously enriched either in real time or in a data warehouse.
Why businesses value data enrichment?
Delivering high-quality and complete data can be a challenge for businesses. There are three types of data enrichment that are most common.
- Behavioural: The enrichment of behavioural data adds customer social and interaction patterns to their other profile data. This allows the identification of areas of interest and a wider context to their actions. Behaviour data can support sales and marketing activity and spend.
- Demographic: The enrichment of demographic data allows the targeting of particular groups of customers, organisations, businesses or employee groups, etc. Messaging can be customised through the enrichment of demographic data.
- Geographic: The enrichment of geographic data allows data to include location specific attributes. This can include address information as well as IP addresses and associated geographic data.
In general, case studies are based on the linking of defined reference metadata hierarchies and taxonomies which allows the standardisation of references to items or concepts in relation to a taxonomic order. For example, grouping together terms such as beak, feathers, pigeon, twitching and Toucan in a refined, improved, and enhanced bird data grouping.
A common use case is the enrichment of customer data an organisation already possesses so it can make more informed decisions. In this case customer data is merged with generally available data online or data received from external sources on any given prospect with the data they have already got in their CRM software. This creates a unified source that's more valuable and useful than its constituents. For example, combining customer information obtained from sales calls with demographic and geographic data.
A similar example is the use of chatbots on websites built on computing and machine learning technologies. Chatbots use enriched data to answer customer’s questions more efficiently and acquire feedback from their discussions with customers.
Enrichment is used in successful marketing campaigns to provide proper customer segmentation, categorizing an audience to deliver more personalized goods and services. Data enrichment improves this task by providing valuable information regarding customers that companies can use to segment them. Segmentation allows a more personal experience for customers and anticipating their needs. Customers want customized and personalized services. It makes them feel special. According to set requirements, data enrichment automation standardizes values, focusing on the most meaningful impact on a target set of customers.
About Finworks
Finworks has years of experience in creating and implementing data quality frameworks for clients. Finworks Data Fabric ensures data is fit and ready to use when and where it is needed and in the correct form for onward consumption.
We provide extraordinary software and service, which enable our customers to continuously gather, manage, question and learn from all the data available to them - and make ongoing improvements to their businesses as a result. Learn more about Finworks services and Finworks Data Management Software.