Data Quality Management – The 5 Pillars Explained
In our highly connected world, there are thought to be several versions of the truth. Even if one is dealing with a single source of the truth, it changes over time. This can be further complicated when there are several sources, which contain snippets of the truth or half-truths:
½ truth + ½ truth = whole truth?
In recent times there has been a belief that one should aim for a Single Version of the Truth (SVoT) when considering Data Management solutions.
This has been primarily driven by the risk of inconsistent, ambiguous, or inaccurate data, commonly referred to as dirty data, which has been estimated at costing the financial industry between 15% -25% in lost revenue, according to Chris Johnson, Senior Manager, ESG Risk, HSBC UK.
In other industries and government departments, it is commonly believed to be far greater. We all remember the data loss incident where a spreadsheet was manually edited and saved, resulting in the reported loss of 16,000 COVID test results.
Was there ever a single version of the truth?
In the early days of computing, a mainframe stood standalone, unconnected to any other devices or the outside world. Each program or computer focused on a specific business domain and it managed its own data. Life was simple then and one can argue that there was a single version of the truth.
As the years have progressed, with the universal adoption of the relational database model, network connectivity, and the success of the Internet, we have seen the proliferation of data. As corporations needed to remain agile to obtain business advances or penetrate new markets, it is not surprising that this resulted in what is known as Multiple Versions of the Truth (MVoT). This does not refer to an alternative version of the facts, but the use of slightly different variants or subsets of the information for related business contexts.
A classic example of this can be seen in the lifecycle of a trade of a financial instrument. As this transaction progresses along the trade lifecycle, different identifiers and specific information for each stage are required. There is an exchange ticker at the initiation of a deal and a settlement identifier in the later stages, with others along the way. Each is considered the truth in their given business domain. In addition, other information is required, such as, to calculate the risk to financial institutions involved in the transaction. There are several business units involved in the workflow and each has its operational interpretation of the truth.
Also, axioms can behave differently within different model representations. A workflow taxonomy comprises multiple steps, these steps are grouped within blocks. Nonetheless, when executing the same workflow constructs their relationship changes, the block now becomes a completion milestone, where the steps within it are expected to be completed before the next block of steps can be initiated.
At this juncture, attempts are underway to construct industry-specific semantic ontologies to support specific business requirements. Existing ontologies include the Global Legal Entity Identifier (Multiple Sectors), Financial Industry Business Ontology (Finance), and SNOMED Clinical Terms (Health). Early adopters are attempting to overlay their Big Data repositories with conceptual semantic graph models with the aim of emulating a single version of the truth. Nonetheless, even the ontologies mentioned above seem to be hit by inconsistent assertions or vagueness in the models. Another observation is that organisations attempt to start the semantic modeling prematurely before all stakeholders are on board.
Naturally, a pragmatic approach is to live with multiple versions of the truth and model this knowledge accordingly, focussing on a specific business context or business domain. An overarching single version of the truth can come after the stakeholders in each business unit have confidence in their model of the truth.
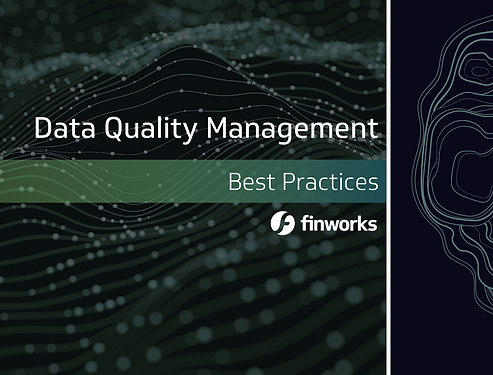
The truth is out there
Maybe if the Federal Bureau of Investigation X-Files Department had adopted the appropriate data quality processes, the TV series may have finished after the initial first six episodes, rather than continue for nine subsequent sessions.
So, let us examine what is understood as Data Quality Management, most believe that this is only achievable if one adheres to its five pillars:
1. Team Composition
The first one that should consider is the knowledge and capabilities of staff. A database designer, for example, will talk in terms of Tables Columns, Primary and Foreign keys. A business analyst or data architect will use terms like classes, objects, and relationships, whilst others within the organisation may use other terminology. One needs to consider who will implement and maintain the solution. Its implementation is only as efficient as the business expertise of individuals. Ideally, one should attempt to adopt an appropriate framework that reduces the need for the involvement of technical staff, such as an IT development team, and remove any potential limitations associated with deploying inappropriate technologies, thus avoiding the risk of the “tail wagging the dog.”
2. Data Profiling
This is the process of examining the data available from the diverse sources. This primarily takes the form of a data discovery function. In a perfect world, this can be automated, by pointing the service at the relevant datasets and it undertakes the first assessment, identifying the data formats, min/max lengths values, and DateTime ranges of data sets to be ingested.
This pillar also examines the metadata required that identify uniqueness and versioning to facilitate the identification of duplication, along with the ability to support bitemporal capabilities between datasets. The latter ensures that queries return the same result for a given historical point and are not influenced by data ingested at a later juncture.
3. Data Quality
This encompasses the creation of an appropriate Data Catalogue containing metadata, and modelling of relationships within and between datasets. Ideally, the data platform should incorporate a Data Quality Management layer comprising both technical and business validation rules, along with the ability to aggregate/consolidate “good” data from several sources. A simple example of a financial data rule is that a user may prefer Bloomberg as the source of debt product reference data, with Refinitiv as the second choice, if not available, and the WM Datenservice for German instruments. Alternatively, financial data could have preferences for reference data from WM Datenservice and analytical data from Bloomberg.
4. Data Reporting
In addition to Data Profiling capabilities, any Data Warehouse solution should incorporate data cleansing activities and data quality logging. This is a prerequisite for the final pillar, Data Resolution, and Repair.
5. Data Resolution and Repair
The framework will need to provide full traceability enabling the user to modify metadata, and workflows, and edit and replay data without the need to always reach out to the originating source.
Truth is in the eye of the owner
It is worth highlighting that it is not just about understanding the truth, in its various forms, but there is also the question, "Can your proposed solution handle the volume?".Not necessarily the volume of information ingested, but its capability to provide enhanced query/response times on large datasets.
The ideal self-service preparation framework should be able to effectively support the Data Quality Management requirements of your organisation, bringing the user's attention to data loss and quality issues. Resulting in reduced dirty data, remediation costs, and ultimately financial implications associated with loss of reputation.
As a final thought, it’s not uncommon for a repository to be shared across several organisations or maintained by a trade association or consortium of associations. The maintenance of shared/communal repositories will be examined in a companion piece with the title ”Considering constructing a shared/communal repository?”.
Martin Sexton is a Senior Business Analyst at Finworks