Building a Robust Healthcare Data Platform
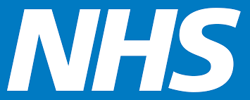
The UK National Health Service (NHS) is the largest nationalised health service in the world. Due to its vast responsibility and the desire to do better for patients, the organisation is always looking into innovative technological solutions.
Data and data-driven innovation could play a role in finding a solution. Several strategies (the national AI strategy for health and adult social care, the data strategy for health and social care), and legislative changes (the Health and Care Bill), are attempting to provide the foundation for such an approach.
Table of Contents
Section 1: Building the largest healthcare data platform
Section 2: NHS solution in delivering care in new and innovative ways
Section 3: Utilising data integration to bring the potential of Integrated Care Systems
Section 4: Healthcare’s data integration challenges
Section 5: A professional approach to managing healthcare data
Section 6: Optimisation and architecture for healthcare big data management
UK’s Desire to Implement a Federated Data Platform (FDP) to Bring its NHS into the digital age
The NHS has accumulated vast amounts of patient data over the years, including extensive logs of visits to hospitals, doctors’ offices, and other medical and social service providers. However, like many other health systems, NHS data is separated into silos, with ownership and management divided among a multitude of organisations within the NHS and the broader care system.
Using AI and data technology advances, the UK government has laid its digital agenda to transform the NHS and social care. The new Federated Data Platform (FDP) is central to the government’s digital agenda and will serve as the foundation for the NHS’s transformation.
The FDP will unify data from 42 Integrated Care Systems, which are groups of healthcare organisations working together to provide seamless medical care. The new federated data platform in the NHS will help bring all this information together to improve efficiency, service quality, and patient outcomes.
What is Federated Data Platform for NHS?
A “data platform” is software that will allow NHS organisations to bring together operational data, which is currently stored in isolated systems, to allow staff to access the data they need in one safe and secure environment, allowing them to coordinate better, plan, and deliver high-quality care.
A “federated” data platform will make it simpler for health and care organisations to collaborate by ensuring that each hospital trust and integrated care system (ICS) has its platform that can collaborate and connect with other data platforms as a “federation.”
Who Will Manage and Build the Data Platform?
The UK government has started a competition to find experts who will construct and manage the platform for its first five years for £360 million ($430 million). According to the FT, consortium members include Black Pear, Eclipse, and Voror Health Technologies, among others. The consortium claims it can deliver the system at a lower cost while protecting patients’ privacy in the UK and reinvesting profits in healthcare.
Data analytics firm Palantir, based in the United States, is believed to be a frontrunner. Palantir is responsible for the Covid-19 data platform in the UK (the Palantir Foundry software), which will be integrated into the new system. The company has a history of developing and maintaining predictive policing software and assisting the NSA and GCHQ to “spy on the whole world” by developing tools that have strengthened global surveillance initiatives.
Most businesses rely on data, and data management enables companies to better organise and access information collected across various sources. However, organising that data in a centralised manner can sometimes be difficult. Creating a data management strategy is essential for companies looking to maintain a competitive advantage and improve internal business operations.
Data management platforms make data management more manageable by automating some of the most time-consuming aspects of unifying and reviewing key data. These systems include databases and analytics tools that enable businesses to store and organise important data to meet the overall data needs of an enterprise.
Importance of Data Integration in NHS
The term “data integration" describes combining data from various locations into one cohesive whole. Data cleansing and transformation occur during the data integration process to guarantee precise analysis. Integrating data from multiple sources allows for generating actionable insights for an organisation.
The healthcare sector produces vast quantities of data, estimated at billions of terabytes per year. Primary sources of patient information include Electronic Health Records (EHRs) and Electronic Medical Records (EMRs). Most of this information can be found online in a readily usable and organised database.
However, there are secondary data sources, such as telemedicine and insurance systems, pharmacy management systems, patient portals, and more. The real challenge is to ingest, process, consolidate, analyse, and then generate timely insights.
What are data integration challenges?
Data is currently oriented around diverse domains rather than the patient who sits at the centre of the landscape due to the wide variety of systems and solutions currently deployed across NHS and ICSs organisations.
The issue with such a framework is that it often requires data translation and team transfer. There is always some loss of value whenever data is translated. It’s a difficult procedure that causes bottlenecks and lowers the quality of the insights gained from the data. This results in extra effort, higher costs, and a higher potential for clinical risks.
Barriers to data sharing, often needed to prioritise security and confidentiality, make it difficult for data to be integrated and, ultimately, for healthcare providers to completely understand their patients:
- Data formats cannot be standardised.
- Challenges in the use of data streams from medical devices.
- Data privacy and compliance regulations.
- Limited data integration processing power.
- Data scientists are not typical end users.
This case study demonstrates four main points of interest:
The implementation, underpinned by Finworks technology, is arguably one of the largest and most complex data-centric systems operating in Europe
The initiative has enabled the financial institution since 2007 to meet its European Directives and achieve financial market stability
By adopting innovative design, technology and security, the operating costs have decreased over time
The collaborative partnership between the financial institution and Finworks has enabled the system to evolve over fifteen years, adapting to change incrementally and with minimal disruption
Why is technology sovereignty important?
For a long time, the UK failed to recognise how much it relied on other countries for technological advancement. There are two potential ways to achieve technological sovereignty in the UK:
1. Complete independence:
This involves excluding foreign actors from the UK market and rebuilding digital infrastructures from scratch, leading to complete independence from foreign services and infrastructures.
2. Acknowledging dependencies:
This model recognises the dependencies between foreign actors and the UK. It aims to promote the UK's competencies while preserving its freedom of choice. The objective is to ensure that existing services can be used to foster economic development while encouraging both investment and innovation. This will lead to competitive regulatory advantages in areas such as AI, data, and digital competition.
We believe that the second model is more inspiring and realistic. To succeed with this model, we recommend focusing on four priorities which complement the NHS Long Term Plan:
1. Accelerating digitalisation:
This involves increasing demand for digital services and developing cloud-native services to be the main source of value in the future of NHS.
2. The commodity of digital infrastructures:
We suggest commodifying digital infrastructures, such as cloud computing, to ensure that NHS have freedom of choice while leveraging innovative services.
3. Emphasising scale:
It is important to emphasise the significance of scale in the digital sector to allow healthcare organisations to reach the necessary size to process big data.
4. Prioritising sovereignty questions:
We recommend creating a process to identify the areas that pose the biggest challenges to sovereignty in healthcare data.
Guide in Building NHS Data Platform
Healthcare organisations can make meaningful use of their data assets if they take a professional approach to managing their data. Financial and operational performance analytics can be enhanced, making it simpler to identify the best practices within the network professionals and implement those across the facility to boost the quality of care provided to patients.
By utilising the healthcare analytics platform, you can reduce the resources required for health systems managers to prepare the metrics and KPIs that drive decisions. In addition, the analytics platform provides a comprehensive view of the processes required to provide healthcare providers with insights, such as the following:
- Harmonising data types specific to healthcare.
- Creating cohort definitions, clinical measurement definitions, and dashboards that are portable.
- Providing relevant datasets for analytical integration.
- Secure exchange of subsets of aggregated data with others.
The following are the primary features of the healthcare data platform:
- A set of tools for enhancing and harmonising clinical and operational data.
- AI and machine learning (ML) tools for analytics and research.
- Ingestion of healthcare-specific data types— and Digital Imaging and Communications in Medicine (DICOM), Fast Healthcare Interoperability Resources (FHIR) in raw form, without the need for parsing, indexing, or data management.
- Open source technology that ingests and harmonises data on-premises, in multi-cloud environments, with minimal code changes and
community support. - Fast, scalable analytics that supports data queries with standard analytics tools and streamlines the visualisation creation process.
- Automation of data lineage and indexing, pipelines for standard data models, and scalability reduce the need for management while lowering
overhead. - Secure data storage is enabled by default through data encryption at rest, EU General Data Protection Regulation (GDPR), information
governance and legal frameworks such as the NHS Act 2006, the Health and Social Care Act 2012, the Data Protection Act, and the Human Rights
Act and other features.
Optimising healthcare data management
With the introduction of electronic health records, organisations' focus has shifted to securely and easily storing and managing patient data.
Numerous data management solutions are available, ranging from simple file storage to more sophisticated enterprise-wide systems. The solution chosen is determined by the organisation's needs and the volume of data to be managed.
Several important considerations must be made as your healthcare organisation moves towards adopting big data, including the following:
Security:
Patient data must be protected against unauthorised access and theft. Healthcare organisations must have policies and procedures in place to manage data security.
Compliance:
Governing agencies establish standards for electronic health records through regulations. These evolve with technology over time, so any cloud provider must be able to meet these specific requirements. Information Governance is a framework for handling personal information in a confidential and secure manner to appropriate ethical and quality standards in a modern health service.
Scalability:
Scalability is critical for any machine learning and artificial intelligence initiatives to proactively identify health issues or assist with research patterns, in addition to usability and availability.
Availability:
As electronic health records connect externally and internally, availability becomes one of the top priorities. Data records will need to be accessed by providers, patients via their phone apps, insurance employees, and others. A solid redundancy and backup plan must be implemented to ensure availability.
A seamless data architecture for managing healthcare data
Several components can be categorised into common layers with a particular function.
Data Sources:
This layer contains various data platform sources. This can include any information system, such as national data sets or collections, ERP or CRM systems, and other open data sources. Data can be supplemented with Excel files, Text files, images, audio, video, or streaming sources, such as IOT devices.
Data Integration Layer:
The responsibility of the ingestion layer is to load data from data sources onto the data platform. This layer is responsible for extracting data from source systems, validating data quality, and storing data in the platform’s landing point or staging area.
Data Processing Layer:
The function of the processing layer is to transform the data to be stored in the correct data model. Depending on the data source type and availability requirements, processing can be performed in batches (scheduled at a specific time/day) or in real time.
Data Storage Layer:
The storage layer takes charge of storing the data. This could be a relational database or another type of storage technology, such as cloud storage, Hadoop, NoSQL database, or Graph database.
Data Analytics Layer:
In the analytics layer, data undergoes additional processing (analysis). This can include a wide variety of (advanced) analytics algorithms, such as those used for machine learning. The results of the analytics can either be sent to the visualisation layer or saved in the storage layer.
Data Visualisation Layer:
The end-user is presented with the data in the visualisation layer. This can take the form of reports, dashboards, self-service BI tools, or APIs so that other systems can utilise the data.
Data Security:
A data platform's essential function is ensuring that only authorised users can access the data. A common method is user authentication and authorisation, but it can also require data encryption (during storage and transfer) and that all activities on the data are audited so that it is clear who accessed or modified which data.
Data Governance:
Data governance involves locating the data in a data catalogue, collecting and storing metadata about the data, managing the master data and/or reference data, and providing insight into the origin of the data in the data platform (i.e., data lineage).
Finworks Data Strategy and Modern Data Platform
There are many ways in which the NHS could benefit from implementing a data integration strategy. Metadata management ensures data accuracy and consistency by integrating data from disparate sources and systems and subsequently classifying, cataloguing, and visualising all data sets. Organisations can save time and improve data quality by automating processes and eliminating manual tasks to augment information made possible through data integration from multiple sources.
Data from a wider variety of sources can allow NHS organisations to visualise their patients, operations, and outcomes, allowing them to improve care and make better decisions about resources. Developing a successful data strategy may involve a number of steps, which explain below;
- Determine which internal and external data repositories will be connected to the platform. Data from partners or customers may be included
here, as well as data from transactions, operations, public data sets, events, sensors, and similar sources. - Specify the types of data, update frequency, and data transformation and cleansing that will be required to successfully integrate the identified
data sources. - Consider the volume and complexity of the data, the need for data processing and analytics capabilities, and the desired level of integration
when deciding on a data integration strategy. Tools such as ETL, ELT, data lakes, data warehouses, and application programming interfaces (APIs)
are all possible alternatives. - Plan the data integration architecture, including the infrastructure that will be required to support the flow of data between applications,
systems, and source endpoints. Data modelling (logical and physical) and selecting suitable data storage and processing technologies may be
required. - To ensure the data integration solution is functioning as intended and meeting the defined requirements, it must be implemented and tested.
Maintaining an efficient and effective data integration process requires constant monitoring and fine-tuning. Rather than waiting for executions to finish, you can adjust data flows as you go along. Your Service Level Agreements (SLAs) and use case will determine the best action.
A Unified Data Platform to Integrate Data and Data Management Processes
You can easily integrate healthcare data without having to write any code at all using Finworks Data Fabric, an end-to-end, modern data integration platform. By implementing Data Fabric, you're able to:
- Data can be extracted from various sources, manipulated to meet your needs, and loaded into an intended system.
- Integrate with external services and databases without writing any code.
- Use data quality rules and advanced profiling capabilities to validate healthcare data.
- Encrypt data and implement role-based access controls to ensure compliance with standard regulations.
- Utilise scheduling and job orchestration capabilities to automate data integration tasks.
Finworks experts can also help lay out technical best practices for healthcare organisations that can improve their data operations with the help of a tailored strategy.